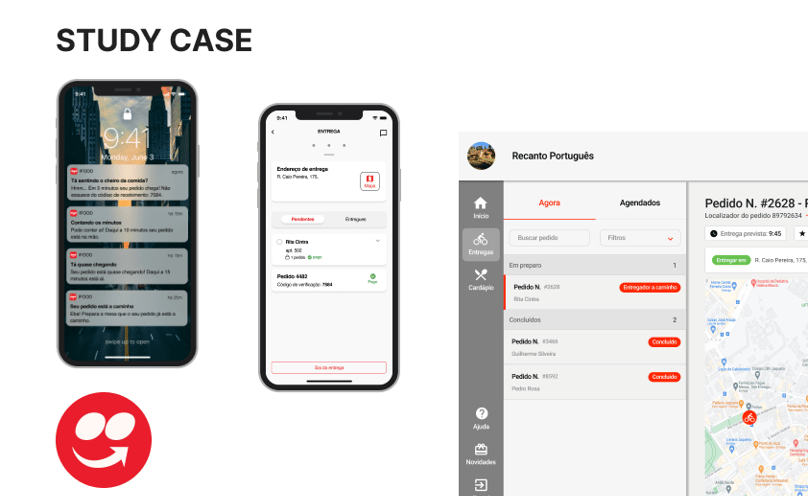
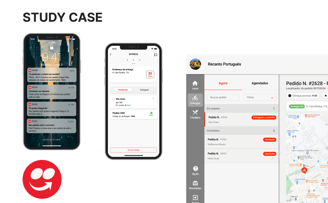
Case study completed in September/2021
THE PROBLEM
Customers, couriers and restaurants suffer from a lack of trust in the delivery system for food delivery apps. When the company is notified that a delivery has not been made, it is difficult to establish what the exact problem was, and whether the problem actually existed.
Customers contact the restaurants saying that the food did not arrive, but the delivery person confirmed the delivery. Who does the company trust? Is the GPS proof enough that the delivery has taken place? What if the delivery person is hired by the restaurant? How can the restaurant and the company be sure of the cause of the problem? And how to avoid those problems?
THE OBJECTIVE
Make the delivery process safer for everyone, preventing financial losses.
THE SOLUTION
Create a feature that both the customer and the delivery person need to confirm in order to complete the delivery, ensuring whether the delivery was actually made or not, and eliminating false or bad faith claims.
MY ROLE
Research
User interviews
Usability Testing
Prototyping
UI Design
UI
THE APP
Ifood is a Brazilian delivery app, specialized in food and groceries. The restaurant has their menu on the app, where the customer can access it and order what they want. Ifood contacts the restaurant with the order, and sends a delivery person to pick it up and take it to the customer. The restaurant is responsible for accepting the order, preparing it, and giving it to the delivery person. They have a chat option to talk to the customer, if necessary, and a phone number for the delivery person. There is no money transaction between the restaurant and the delivery person, or the customer. The customer pays through the app, and the app sends the money to the restaurant after a period of time, with the fees already discounted.
Ifood is currently the most used delivery app in Brazil, by a large margin, according to research. It is available in over 100 cities, and, according to research, Ifood dominates 83% of the market, with the second place (Uber Eats) at 13%.
THE RESEARCH
Phase 1: Empathize
In this first phase of the research, I created 3 forms to be answered by customers, couriers and restaurants signed up in the app. Forms were posted to specific Facebook groups, and responded to anonymously; I also conducted interviews through Whatsapp and in person, using the form as a basis. The main purpose of these forms was to identify users’ pain points, and find a common denominator on which to focus the next phases of the research.
Phase 2: Define
Customers:
For users who place orders in the app, most feel insecure about the delivery person’s location. Delivery people hired by the restaurant are not tracked when delivering; the app’s own delivery people are tracked, but users say the location is not accurate, or updated in real-time.
Delivery people:
For delivery people, there are cases of customers lying that the order has not been delivered, and people taking an order that doesn't belong to them. The penalty is a 48-hour ban in the app, which, depending on the days of the week, can generate a loss of more than R$1,000.00 (around $250 american dollars). Deliverers feel as if they are at the mercy of customers and the app, and confirm that the current delivery system does not protect them in cases of fraud.
Restaurants:
For restaurants registered in the app, the location of the delivery person is also inaccurate or even inexistent. They feel more secure when hiring their own delivery people, but the cost is not worth it if the volume of deliveries is low. With app deliverers, the instruction the restaurants receive is that, once they deliver the order to the courier, they are no longer responsible for the order. However, when there are problems with delivery, customers usually want the restaurant to solve it, and if it is not resolved, they won't order from the restaurant again, but will continue to use the app.
Phase 3: Ideate
From the information collected during Phase 2, I gathered and classified common points mentioned by the interviewees, identified possible improvements, and created three personas and their respective journeys to represent the three users of the app. With those in hand, I could better understand the goals and frustrations of users.
Customers, Delivery People and Restaurants:
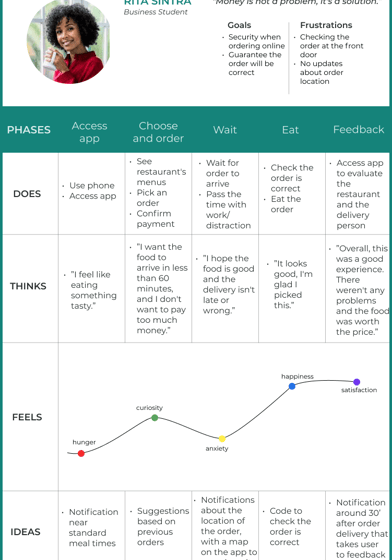
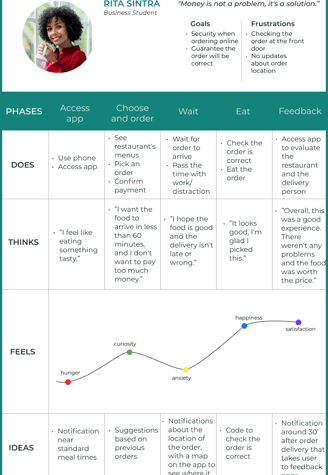
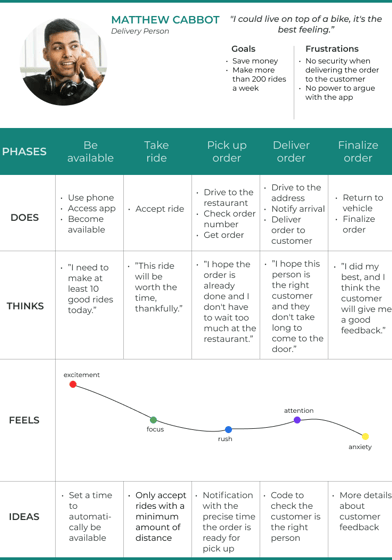
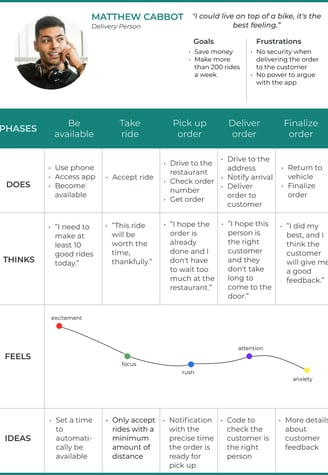
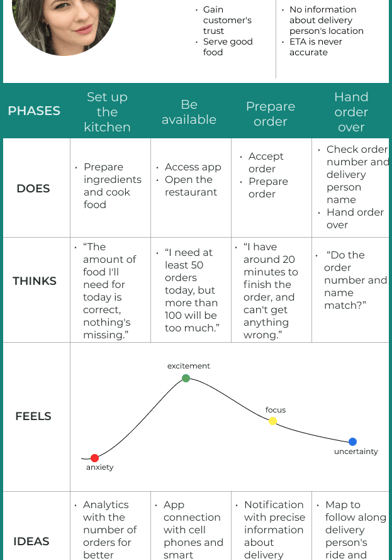
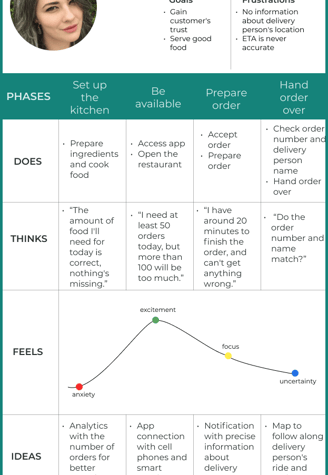
Phase 4: Prototype
Prototyping was carried out based on testimonies and images shared by the interviewees, as only delivery people and restaurants have access to their respective platforms. Therefore, the prototypes are based on images taken from the internet.
Customers:
The simplest change from a technical point of view, the customer would receive a 4-digit code that must be informed during delivery. In addition, they would receive notifications about the real-time location of the delivery person.
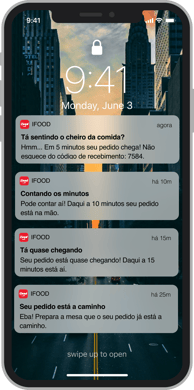
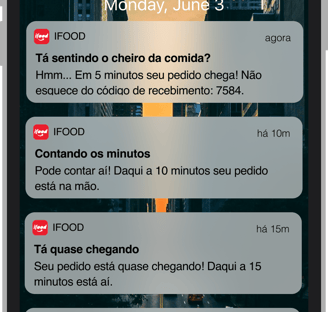
Delivery people:
When delivering the product, the delivery person will confirm the code that the customer informed them in a text field, and the delivery can only be completed if the code is correctly informed.
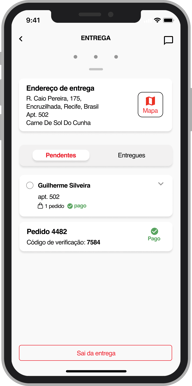
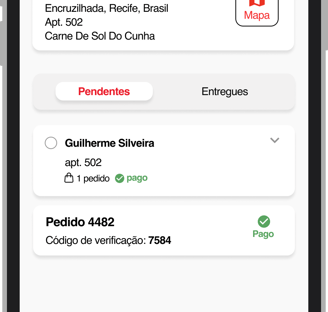
Restaurants:
A map will indicate the location of the delivery person, and it will be updated in real time from the moment the delivery person accepts the race. From this map, restaurants will receive notifications of the delivery person’s location and can more accurately plan for the order to be ready as soon as the deliverer arrives. Then, they will be able to follow the path that the delivery person takes, until the delivery is completed.
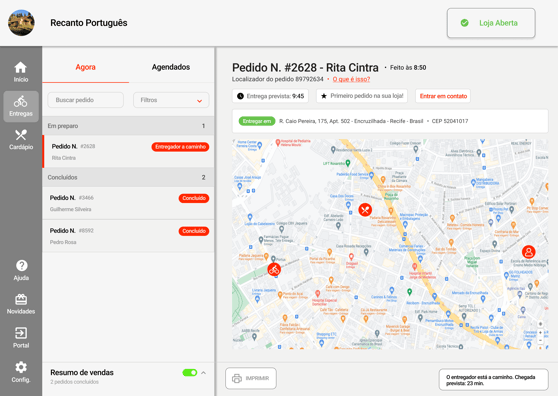
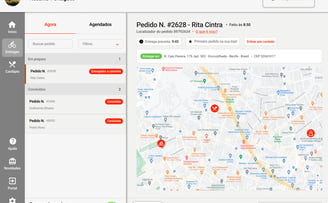
Phase 5: Test
Customers reported ease and familiarity with the new functionality. Some have reported that it already exists in other similar apps. Notifications are especially useful for users who live in houses, or buildings without a doorman: they allow for better time management, avoiding delays in picking up the order with the delivery person.
Customers:
Ideally, the tests would be done with the same people who were interviewed in phase 1, but I couldn’t get in touch with everyone, especially the delivery people. Therefore, the tests were done with new participants. As the changes were simple, there were no problems regarding the understanding and usability of the new features.
“Very simple, there’s no secret.”
“I can place the order and chill until the app lets you know that the biker is close.”
Delivery people:
Deliverers reported the same as customers. Furthermore, they said the extra level of security makes them more comfortable working with the app.
“It’s what we need.”
“It doesn’t take any work and it guarantees that the order went to the right person.”
Restaurants:
Restaurants reported greater control when preparing food, preventing the order from waiting too long for the delivery person, which lowers the quality of the food and, consequently, the customer experience and the restaurant’s overall score on the app.
“It would be great, so the food doesn’t arrive cold for the customer.”
“It’s great, if the biker is far away, we’ll hold the order until he gets closer.”
NEXT STEPS
#now
Survey with a larger number of participants, adding other metrics such as: average order volume, average ticket, financial loss due to specific delivery problems, among others.
#next
Implementation of new features in beta mode, with selected users, to monitor metrics on a smaller scale, and identify possible technical errors.
#later
Implementation of new features, and monitoring of metrics.
Update September/2021
A large party of this case study was carried out between September and October 2020. In September 2021, some of the features described in this case study were implemented by Ifood, such as the delivery code.
Restaurants now also have access to a map with the location of the delivery person. In addition, a button has been implemented to notify the delivery person when the order is ready.